The Key Applications of AI and ML in Data Analysis
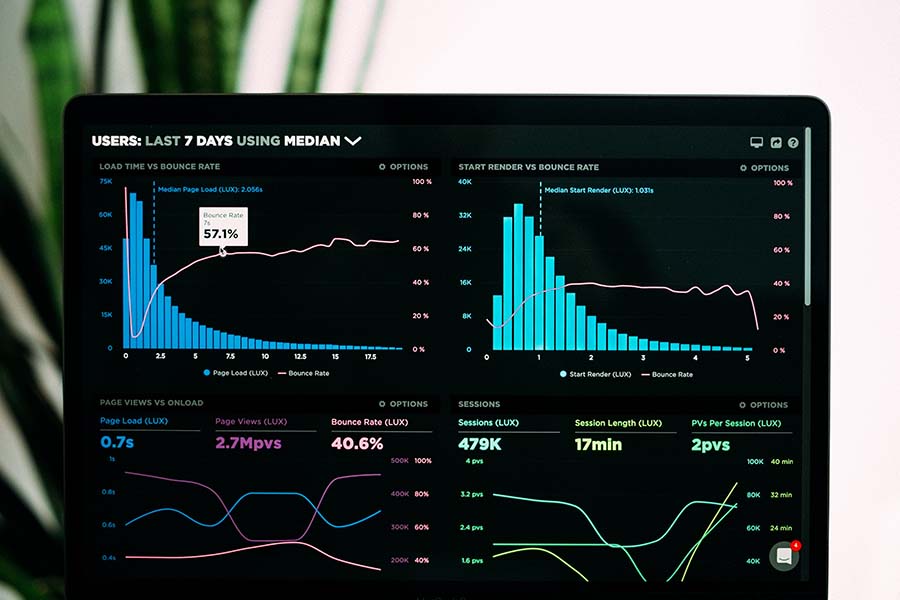
Amidst the swift progress of technology, Artificial Intelligence (AI) and Machine Learning (ML) have emerged as prominent players across diverse industries. Data analysis stands out as a domain where these cutting-edge technologies demonstrate immense potential.
This blog delves into the pivotal applications of AI and ML in data analysis, showcasing their transformative impact on extracting meaningful insights from extensive data sets.
Introduction to AI and ML in Data Analysis
Data analysis examines, cleans, transforms, and models data to discover useful information, draw conclusions, and support decision-making. The traditional methods of data analysis often involve manual efforts and are time-consuming. However, AI and ML have proven to be game-changers in this field by automating processes, improving accuracy, and uncovering patterns that may have otherwise gone unnoticed.
More accessible and Faster Data Preparation
One of the most time-consuming tasks in data analysis is data preparation. AI and ML algorithms can significantly reduce this burden by automating data cleaning, formatting, and integration tasks. These technologies can handle high volumes of data, allowing them to transform raw data into a clean and structured format. By automating the data preparation process, AI and ML ensure accuracy and efficiency in the analysis process.
AI and ML algorithms can automate data cleaning tasks such as removing duplicate entries and correcting errors, saving analysts valuable time.
These technologies can also automate the formatting of data, ensuring consistency and compatibility across different datasets.
Data integration from various sources can be automated, eliminating the need for manual data consolidation and reducing the risk of human error.
Predictive Analytics
Predictive analytics is a powerful application of AI and ML in data analysis. Using historical data and statistical algorithms, these technologies can predict future trends, behaviours, and outcomes. This helps businesses make informed decisions, identify potential risks, and optimise their strategies. Whether forecasting sales, predicting customer churn, or anticipating market trends, AI and ML enable businesses to gain a competitive edge by leveraging data-driven insights.
AI and ML algorithms analyse historical data to identify patterns and correlations, enabling accurate predictions.
These technologies can identify key factors contributing to specific outcomes, helping businesses understand the drivers behind their success or failure.
AI and ML models can adapt and improve their predictions by continuously learning from new data, ensuring up-to-date insights.
Anomaly Detection
Detecting anomalies or outliers in large datasets is a challenging task. However, AI and ML techniques excel in anomaly detection by leveraging pattern recognition and statistical models. By learning from historical data, these technologies can identify abnormal patterns, outliers, or suspicious activities that may indicate fraud, system malfunctions, or cybersecurity threats. This enables businesses to proactively address potential risks and ensure the integrity and security of their data.
AI and ML algorithms can identify unusual patterns in data that may indicate fraudulent activities, such as unauthorised access or suspicious transactions.
Through constant data stream monitoring, these technologies swiftly identify anomalies and trigger alerts, empowering businesses to respond promptly. The application of AI and ML in anomaly detection extends to vital sectors like finance, healthcare, and cybersecurity, fortifying critical systems and thwarting potential threats.
Natural Language Processing (NLP)
Natural Language Processing (NLP) is an AI subfield focusing on the interaction between computers and human language. In data analysis, NLP techniques allow for extracting valuable insights from unstructured data sources such as text documents, social media posts, or customer reviews. By analysing sentiment, identifying key topics, and extracting relevant information, NLP enables businesses to gain a deeper understanding and enhance decision-making based on textual data.
NLP algorithms can analyse the sentiment expressed in customer reviews, helping businesses gauge customer satisfaction and identify areas for improvement.
By extracting key topics from text documents, NLP enables businesses to categorise and organise unstructured data, making it easier to analyse and draw insights.
NLP techniques can also be applied to social media data, allowing businesses to monitor brand perception, identify trends, and engage with customers more effectively.
Recommendation Systems
Recommendation systems have become integral to various online platforms, providing personalised suggestions to users based on their preferences and behaviours. AI and ML techniques power these systems by analysing user data, past interactions, and patterns to make accurate recommendations. Whether it’s suggesting products, movies, or articles, recommendation systems enhance user experience, increase engagement, and drive revenue by delivering tailored content to individual users.
AI and ML algorithms analyse user behaviour and preferences to understand their unique preferences and interests.
These technologies can identify user patterns and similarities, allowing them to make accurate recommendations based on collective user data.
Recommendation systems continuously learn and adapt based on user feedback, ensuring the recommendations remain relevant and personalised.
Image and Video Analysis
Through computer vision techniques, AI and ML have revolutionised visual data analysis, such as images and videos. By training algorithms on massive datasets, these technologies can recognise objects, faces, emotions, and other visual attributes. This has applications in various industries, including healthcare (diagnosis from medical images), security (surveillance and facial recognition), and e-commerce (visual search and product recommendation).
AI and ML algorithms can analyse medical images to assist in the diagnosis of diseases, enabling early detection and improved patient outcomes.
Surveillance systems can use computer vision techniques to identify individuals, track their movements, and detect suspicious activities.
In e-commerce, AI and ML enable visual search, allowing users to find products by uploading images, enhancing the shopping experience, and improving conversion rates.
Data Clustering and Segmentation
Clustering and segmentation techniques are essential in organising and categorising data into meaningful groups. AI and ML algorithms can automatically identify similar patterns, group related data points, and segment datasets based on various attributes. This enables businesses to gain insights into customer segmentation, market segmentation, or identifying patterns in large datasets, leading to more targeted strategies and improved decision-making.
AI and ML algorithms can cluster customer data based on purchasing behaviour, demographic information, or other relevant attributes, enabling businesses to tailor their marketing strategies to specific customer segments.
These technologies can segment market data to identify customer segments, allowing businesses to develop targeted marketing campaigns and personalised offerings.
In large datasets, clustering and segmentation techniques can uncover hidden patterns or relationships among data points, providing valuable insights for business strategy and decision-making.
Automation and Process Optimisation
AI and ML can automate repetitive and mundane data analysis tasks, freeing analysts’ time to focus on more complex tasks. By leveraging algorithms that learn from historical data, these technologies can identify patterns, make predictions, and suggest optimal solutions. From automating data cleansing to optimising supply chain processes, AI and ML streamline operations, improve efficiency, and drive productivity in data-driven organisations.
AI and ML algorithms can automate the process of data cleansing, ensuring data accuracy and consistency.
These technologies can optimise supply chain processes by analysing historical data, identifying bottlenecks, and suggesting improvements.
By automating repetitive tasks, AI and ML enable analysts to allocate their time and resources more effectively, focusing on tasks that require human expertise and creativity.
Conclusion
AI and ML have brought significant advancements to data analysis, revolutionising how businesses extract insights from massive amounts of data. From automating data preparation to predictive analytics, anomaly detection, NLP, recommendation systems, image/video analysis, clustering, and automation, these technologies offer endless possibilities for businesses to gain a competitive edge. As AI and ML continue to evolve, the applications in data analysis are only expected to grow, empowering organisations to make data-driven decisions and uncover hidden opportunities.
Be sure to check out our other related posts if you enjoyed this one:
- The Rise of Collaborative Robots: Transforming Industries
- Unmasking Cyber Secrets: The Art of Deception Revealed!
- Decoding Cyber Threats: The Social Engineering Menace
- Unlock the Ultimate Quest: Ready Player One’s Audio Adventure!
- Revolutionising Wellness: Metaverse Therapy Unleashes Mental Liberation!
- Code Mastery Unleashed: Transform Your Skills with Clean Code by Robert C. Martin! 🚀
- Top Must-Have Tech Gadgets for Kids – Unbelievable Fun!
- Mastering Crypto Trading: Proven Strategies
- Unveiling Ethereum 2.0: Advancements & Impact
- AI Transforms E-Commerce: A Digital Revolution
Sign up for updates on this blog and our latest posts if you enjoyed reading this one.
FAQ
How can AI and ML algorithms automate data preparation in data analysis?
AI and ML algorithms can automate data cleaning tasks such as removing duplicate entries and correcting errors, saving analysts valuable time.
These technologies can also automate data formatting, ensuring consistency and compatibility across different datasets.
Data integration from various sources can be automated, eliminating the need for manual data consolidation and reducing the risk of human error.
How do AI and ML enable predictive analytics in data analysis?
AI and ML algorithms analyse historical data to identify patterns and correlations, enabling accurate predictions.
These technologies can identify key factors contributing to specific outcomes, helping businesses understand the drivers behind their success or failure.
AI and ML models can adapt and improve their predictions by continuously learning from new data, ensuring up-to-date insights.
How do AI and ML excel in anomaly detection in data analysis?
AI and ML algorithms can identify unusual patterns in data that may indicate fraudulent activities, such as unauthorised access or suspicious transactions.
By continuously monitoring data streams, these technologies can quickly detect anomalies and trigger alerts, allowing businesses to take immediate action.
Anomaly detection using AI and ML can be applied to various industries, including finance, healthcare, and cybersecurity, to safeguard critical systems and prevent potential threats.
What role does NLP play in data analysis?
NLP algorithms can analyse the sentiment expressed in customer reviews, helping businesses gauge customer satisfaction and identify areas for improvement.
By extracting key topics from text documents, NLP enables businesses to categorise and organise unstructured data, making it easier to analyse and draw insights.
NLP techniques can also be applied to social media data, allowing businesses to monitor brand perception, identify trends, and engage with customers more effectively.
Credits
Featured photo by Luke Chesser on Unsplash.